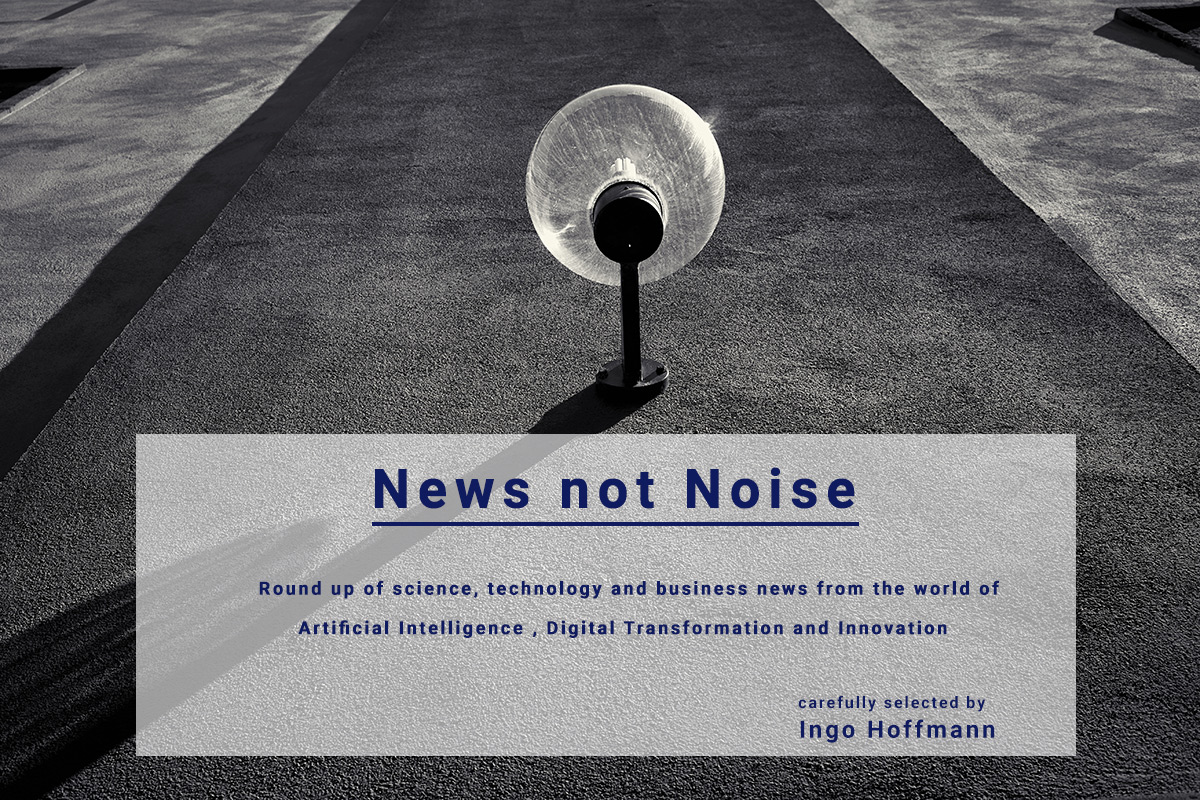
News not Noise – #8
This week’s topics continues the discussion on national AI strategies and the focus on China. We also look at the impact of AI on the future of work and some important aspects of machine learning.
AI Strategy
An Overview of National AI Strategies | Tim Dutton
I have looked at the topic of AI nationalism and national AI strategies in the last newsletter. Tom Dutton has created a great overview of national AI strategies and he is updating it continuously.
The ascent of Artificial Intelligence: How will AI change the nation-state? | Brookings
Different models for the use of data as a key part of national AI strategies. Four models are emerging: data as an asset (USA); Data as a risk (Europe, Japan); Data for state control (China) and Data for inclusion (India). Interesting perspective.
Regulating AI in the era of big tech | The Gradient
Another interesting view on the topics of national AI policies and strategies. Melody Guan argues that on the subject of private and ethical AI, the U.S. government has been disinterested. Other governments have presented AI strategies that highlight the awareness of ethical concerns in AI and the commitment to developing safe and beneficial AI technologies
Facial recognition technology: The need for public regulation and corporate responsibility | Microsoft
Microsoft is adressing a key question: Facial recognition technology raises issues that go to the heart of fundamental human rights protections like privacy and freedom of expression. They are arguing for more government regulations. A very much needed debate not only for facial recognition with no easy answers.
Future of Work
High-Skilled White-Collar Work? Machines Can Do That, Too | The New York Times
The fashion industry as one example how AI and intelligent machines can do creative work , too. But by no means limited to fashion.
The rise of ‘pseudo-AI’: how tech firms quietly use humans to do bots’ work | The Guardian
A look behind the curtains of some „AI“ companies. It is not always what you think it is. It’s hard to build a service powered by artificial intelligence. So hard, in fact, that some startups have worked out it’s cheaper and easier to get humans to behave like robots than it is to get machines to behave like humans.
Machine Learning
Attacks against machine learning — an overview | Elie.net
Good overview of the attacks techniques that target AI systems and how to protect against them.
MLflow: A platform for managing the machine learning lifecycle | O’Reilly
Machine Learning projects can be quite difficult to implement. MLflow is a new open source project to build an open ML platform. Beyond being open source, MLflow is also “open” in the sense that anyone in the organization—or in the open source community—can add new functionality, such as a training algorithm or a deployment tool, that automatically works with the rest of MLflow
China
China’s Artificial Intelligence Revolution: a Sputnik Moment for the West? | The Diplomat
Kris Hartley argues that China’s rise in AI and other technologies will have global economic, social, and diplomatic implications that will resonate for decades. The U.S. and other Western nations must strengthen their ambition to remain competitive.
The devil’s bargain for AI companies working in China | Axios
American tech companies and research institutions — involved in the development of artificial intelligence in both the U.S. and China — face elevated ethical questions as the two superpowers race for dominance in the field. Same is true for Europe.